Introduction
Artificial intelligence (AI) is transforming healthcare across multiple domains, from drug discovery, personalized medicine, and medical diagnostics. In pathology, there is an increasing shift towards digital workflows, with growing evidence that AI-based analysis tools can assist pathologists by improving diagnostic efficiency and inter-rater agreement in clinical biopsy scoring1,2,3. Integrating AI into digital pathology supports a more data-driven, reproducible, and precise approach to cancer diagnostics (Figure 1).
As digital pathology gains traction, there is increasing opportunity for pathologists to leverage AI-based image analysis tools for histopathology evaluation and precise tumor quantification for both clinical and research applications. In the clinical setting, AI can enhance diagnostic accuracy, speed, and consistency for faster case prioritization, while in research, AI enables more accurate quantification of tumor content to support more precise sample selection for downstream analyses. By enhancing histopathology evaluation in both domains, AI is helping to drive significant innovation in pathology.
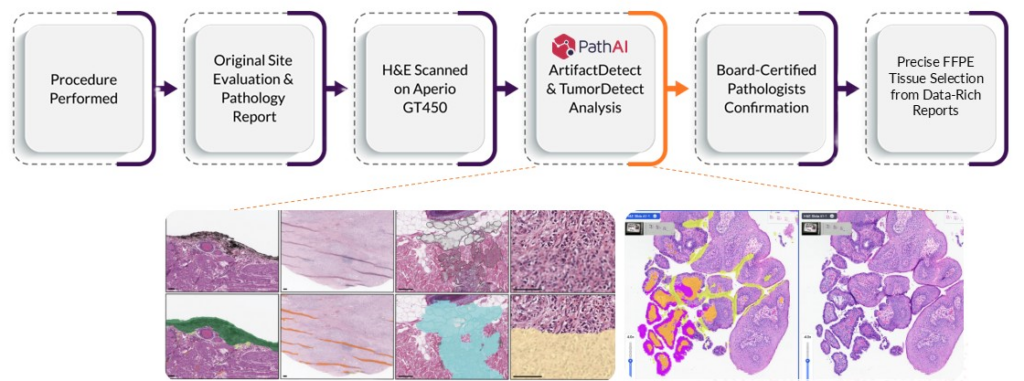
Figure 1. Discovery Life Sciences has partnered with PathAI to deploy AISight® Image Management System (IMS), ArtifactDetect, and TumorDetect products into its biospecimen pathology pipeline. This integration streamlines the workflow, improves tumor detection and quantification across diverse tissue types, and reduces the subjectivity and variability in qualitative pathologist evaluations.
Variability in Tumor Percentage Quantification
When evaluating FFPE (Formalin-Fixed, Paraffin-Embedded) biospecimens for research, pathologists typically assess tumor content by manually examining stained slides under a microscope. This process involves two key steps: distinguishing tumor from non-tumor tissue and estimating the tumor area. While pathologists excel at identifying tumor tissue, visually estimating its size is inherently challenging. As a result, tumor percentage (%) assessments vary significantly between different pathologists, and even across independent reviews. Inconsistent tumor content assessments in the research setting can impact sample suitability for downstream testing.
To quantify this variability, Discovery conducted a study to evaluate tumor % assignments for multiple FFPE tissue samples across three independent pathology reviews (Figure 2).
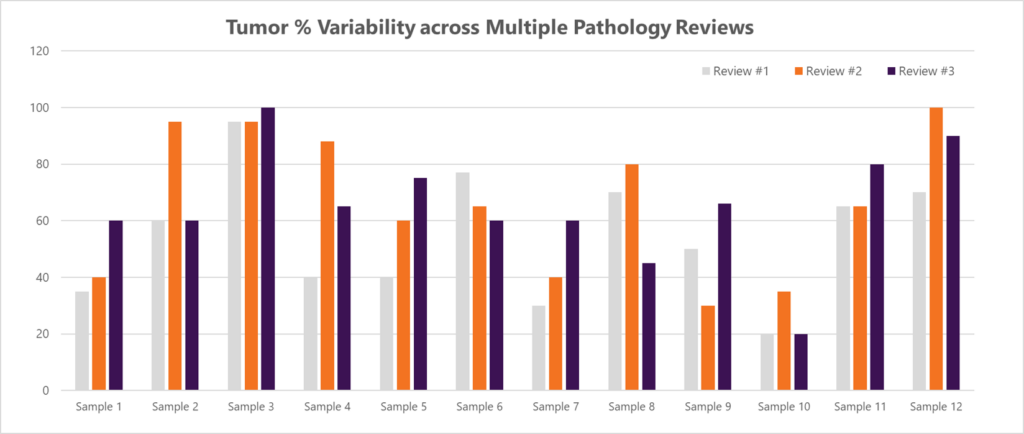
Figure 2. Tumor % assignments for twelve different FFPE tissue samples across three independent pathology reviews.
Linear regression analysis comparing different pathology reviews (Review 1 vs. Review 2, Review 2 vs. Review 3, and Review 1 vs. Review 3) shows lower correlation coefficients (R² value) indicating a lack of agreement and shows the variability in tumor % estimates (Figure 3).
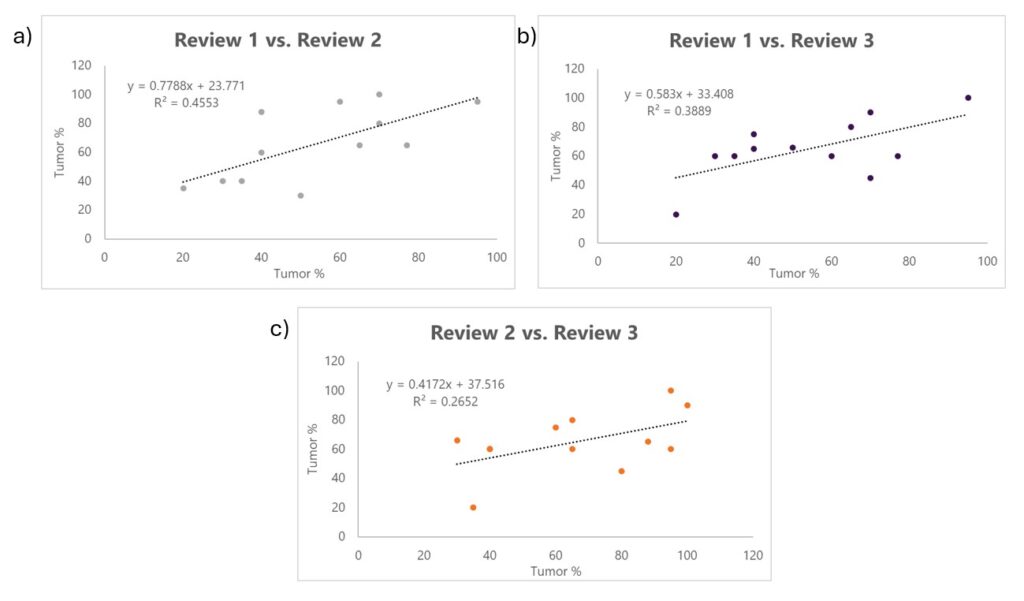
Figure 3. Linear regression analysis comparing tumor percentage estimates between independent pathology reviews. (A) Review 1 vs. Review 2 (R² = 0.4553), (B) Review 2 vs. Review 3 (R² = 0.2652), and (C) Review 1 vs. Review 3 (R² = 0.3889). The lower R² values (<0.5) indicate less agreement and more variability in tumor % assessments across pathologist reviews.
This data confirms the subjectivity and variability of human visual estimation, making a strong case for adopting a more precise approach to tumor quantification.
Reducing Variability in Tumor % Assessments with AI Solutions
Computers excel at precise measurements, but their application for tumor quantification has historically been limited by two key challenges: digitization and tumor recognition. Advances in whole-slide imaging (WSI) and AI-powered tissue annotation have now overcome these barriers, expanding the capabilities of pathology laboratories2.
PathAI’s AISight® Image Management System, and specifically the ArtifactDetect and TumorDetect algorithms from PathAI, have been trained on tens of thousands of images with millions of expert annotations. They can systematically and rapidly analyze digital pathology slides at the pixel level, allowing for faster and more accurate and reproducible assessments.
Quality Control with ArtifactDetect
ArtifactDetect performs an initial QC check on the slide images, identifying and flagging histology and scanning artifacts such as folds, bubbles, and blur. Non-evaluable slides are flagged for replacement to ensure that pathologists spend their time reviewing high-quality evaluable slides (Figure 4).
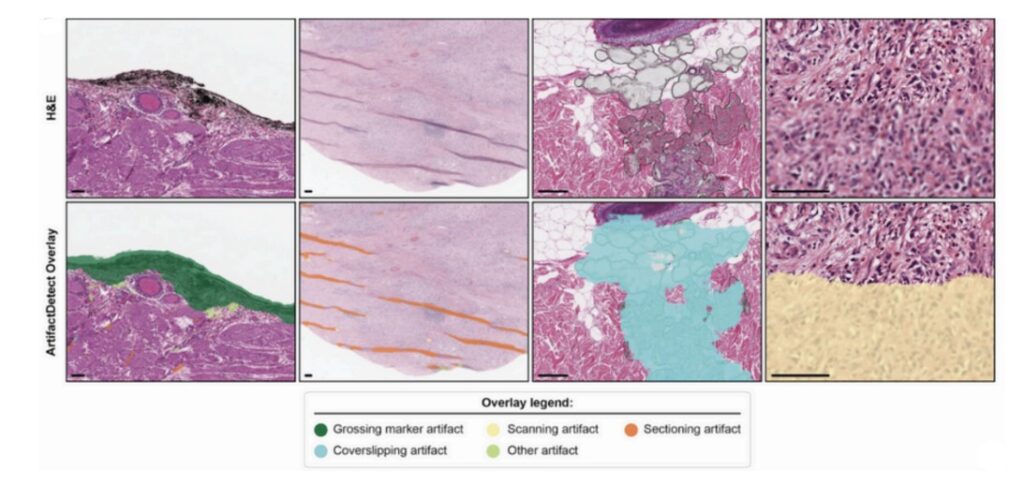
Figure 4. ArtifactDetect overlay identifies and flags artifacts that can interfere with pathologist review.
Precision Tumor Quantification with TumorDetect
TumorDetect brings a new level of accuracy in pathology. Rather than relying on visual estimation of tumor area and tumor %, which we have shown to exhibit significant variability, TumorDetect identifies tumor and classifies subregions as cancer (the tumor epithelium), cancer-associated stroma, and tumor necrosis. Once each pixel has been identified as tumor tissue or non-tumor tissue, the tumor content is calculated as tumor area in mm² and as tumor percentage (tumor % = total tumor pixels/total tissue pixels x 100).
To validate its precision in tumor area quantification, a same-slide concordance study was conducted (Figure 5). Across two re-deployments on the same slide, TumorDetect demonstrated 100% concordance in predicted tumor area to the nearest 0.01 mm² (n = 12). This level of reproducibility confirms that AI-driven tumor quantification is highly consistent, with no variability observed across repeated analyses.
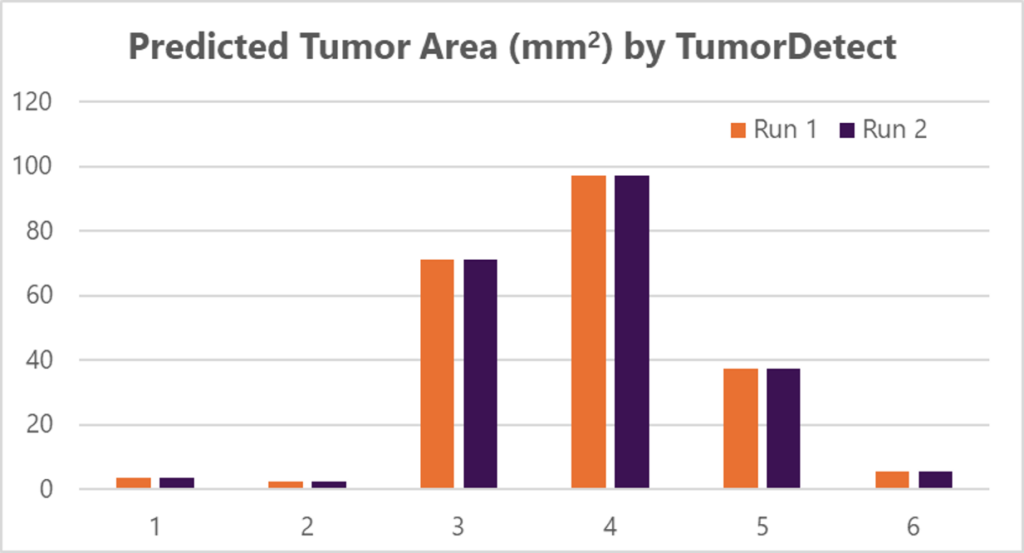
Figure 5. Same-slide concordance analysis of tumor quantification across six different slides over two runs.
TumorDetect can help prioritize pathologists’ caseloads based on tumor content. Slides with potential malignant tumors or other fields of interest (FOI) can be flagged. TumorDetect makes sure pathologists prioritize and assess the “probable cancer” patient cases first. Additionally, the AI algorithm can generate overlay visualizations and slide-level quantifications that aid pathologist review and enable more precise assessments (Figure 6). Together, these features help pathologists expedite case reviews, accelerate the ordering of additional tests, and improve overall case turnaround time.
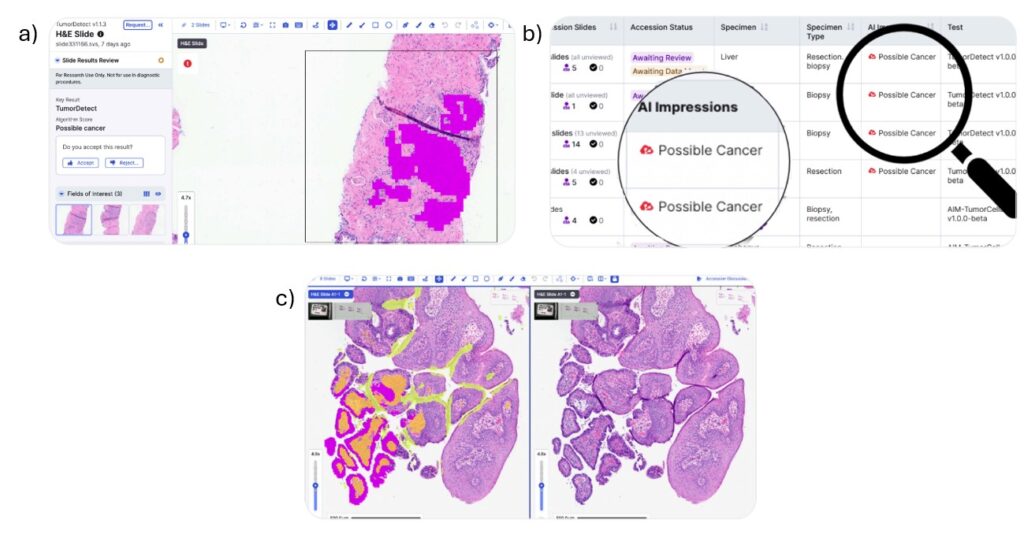
Figure 6. (A) TumorDetect classifies each pixel to provide absolute tumor quantification. (B) It can also flag FOIs for pathologist review enabling faster clinical case prioritization. (C) Overlay visualizations and slide-level quantifications can be generated to aid pathologist review.
Accurate tumor quantification is essential for optimal sample selection in oncology research. Tumor content varies significantly across different FFPE blocks from each surgical case, and human pathologists’ estimates of tumor content can vary significantly, making sample selection difficult. As a result, precise tumor quantification is crucial for selecting the most appropriate samples for downstream evaluations. For instance, in bulk genomic analysis, tumor content directly influences the ability to detect low VAF (Variant Allele Frequency) variants. A higher tumor content improves the sensitivity of detecting rare mutations, which is critical for identifying genetic changes that may drive cancer progression. TumorDetect can provide the precise tumor % and tumor area (mm2) that allow researchers to identify the best samples for their research application.
Conclusion
The last few years have seen tremendous growth in the development and integration of AI tools across multiple industries, and pathology is no exception. These AI-driven innovations are accelerating diagnostic workflows, eliminating human error, improving inter-observer reproducibility, and even providing prognostic predictions3. The integration of PathAI solutions into Discovery’s biospecimen pathology pipeline reinforces our commitment to embracing AI-driven advancements and staying at the forefront of scientific innovation.
The scope of Discovery’s collaboration with PathAI extends beyond AISight® and also includes DiscoverAI, the industry’s first joint offering with PathAI that delivers quantitative characterization of the tumor microenvironment. This advanced, fit-for-purpose AI analysis tool provides human-interpretable features (HIFs) such as cell counts, densities, and spatial relationships across tumor regions, unlocking spatial insights beyond human pattern recognition.
By adopting AI-powered solutions, Discovery is driving a data-first approach that will accelerate the development of new assays and diagnostic tools, and support advancements in drug discovery and personalized medicine, which will contribute to better patient outcomes.
Download Brochure to Learn more about Discovery’s AI-Powered Pathology Solutions
TumorDetect and ArtifactDetect are workflow optimization algorithms available on AISight. AISight® is for Research Use Only in the US; AISight® Dx is CE-IVD marked in the EEA, UK, and Switzerland.
References
- McNeil C, Wong PK, Niranjan Sridhar N, et al. An End-to-End Platform for Digital Pathology Using Hyperspectral Autofluorescence Microscopy and Deep Learning-Based Virtual Histology. Modern Pathol. 2042;37(2):100377. doi: 10.1016/j.modpat.2023.100377
- Shafi S, Parwani AV. Artificial intelligence in diagnostic pathology. Diagn Pathol. 2023;18(1):109. Published 2023 Oct 3. doi:10.1186/s13000-023-01375-z
- Försch S, Klauschen F, Hufnagl P, Roth W. Artificial Intelligence in Pathology. Dtsch Arztebl Int. 2021;118(12):194-204. doi:10.3238/arztebl.m2021.0011